All Tags
AWS
algorithm-design
architecture
cloud-principles
cost-reduction
data-centric
data-compression
data-processing
deployment
design
edge-computing
energy-footprint
hardware
libraries
locality
machine-learning
management
measured
migration
model-optimization
model-training
performance
queries
rebuilding
scaling
services
strategies
template
workloads
Tactic: Monitor Computing Power
Tactic sort:
Awesome Tactic
Type: Architectural Tactic
Category: green-ml-enabled-systems
Title
Monitor Computing Power
Description
Estimating and calculating the energy footprint of a machine learning model can help to reduce the computational power of ML models. Monitoring the energy consumption of a ML model in the long term helps to identify those components where energy is being inefficiently utilized. This can serve as a starting point for making improvements to reduce energy consumption. There has been a lack of easy-to-use tools to do that, but recently researchers have provided frameworks for how to estimate or calculate the energy footprint of machine learning.
Participant
Data Scientist
Related software artifact
Machine Learning Model
Context
General
Software feature
< unknown >
Tactic intent
Improve energy efficiency by monitoring computing power of machine learning in the long term
Target quality attribute
Energy Efficiency
Other related quality attributes
< unknown >
Measured impact
< unknown >
Source
Qingqing Cao, Yash Kumar Lal, Harsh Trivedi, Aruna Balasubramanian, and Niranjan Balasubramanian. 2021. IrEne: Interpretable Energy Prediction for Transformers. Proceedings of the 59th Annual Meeting of the Association for Computational Linguistics and the 11th International Joint Conference on Natural Language Processing (Volume 1: Long Papers) (2021). [DOI](https://doi.org/10.48550/arXiv.2106.01199); Mohit Kumar, Xingzhou Zhang, Liangkai Liu, Yifan Wang, and Weisong Shi. 2020. Energy-Efficient Machine Learning on the Edges. In 2020 IEEE International Parallel and Distributed Processing Symposium Workshops (IPDPSW). 912–921. [DOI](https://doi.org/10.1109/IPDPSW50202.2020.00153)Graphical representation
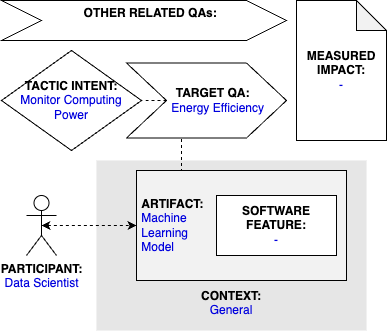